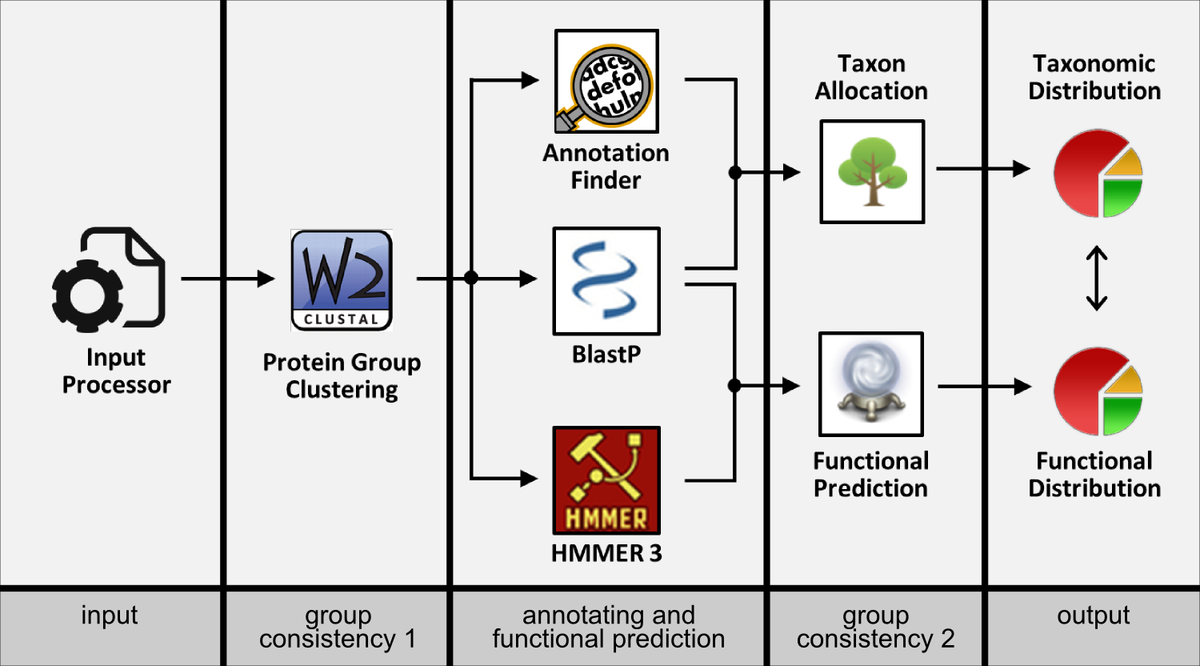
One of the most frequently used approaches in proteomics, called bottom-up or shotgun proteomics, relies on tandem mass spectrometry of peptides after enzymatic protein digestion and subsequent correlation of the obtained spectral data with amino acid sequences of a given protein database (Eng et al., 1994).
The final protein identifications have to be inferred from the resulting peptide spectrum machtes (PSMs). This can be surprisingly difficult since peptides can be shared by many different proteins as conserved motifs (for review see Nesvizhskii and Aebersold, 2005). Especially in metaproteomic analyses where target databases contain naturally many homologous proteins from closely-related organisms the proportion of PSMs that can be assigned to more than one protein is high. To handle this, proteins can be clustered based on the shared PSMs (e.g. described by Koskinen et al., 2011). Each of these clusters (or protein groups) is represented by a master protein which has been selected based on PSM coverage and probability scores. However, the involved algorithms are highly diverse and you should refer to the respective manual to get more information on protein grouping provided by the software you are using.
PSMs sharing proteins belonging to numberless taxonimical origins and involved in various functions. Thus, it is difficult or even impossible to choose a single master protein representing the whole group on taxonimical as well as functional level. With Prophane we provide a fully automatic (but highly adaptable) workflow which is not relying on master protein information but considering all protein members within the protein groups (firstly described in: Schneider et al., 2011). Each group is analyzed regarding commonalities on both taxonimical and functional level between the covered members. Additionally, Prophane eases data inpsection and interpretation by organizing all relevant information and analysis results in intuitive and interactive tables.
More information: www.prophane.de/index.php
Prophane-Link: www.prophane.de